Effectiveness of expert systems in the selection of coffee beans (Coffea arabica)
a systematic review
DOI:
https://doi.org/10.55873/rad.v1i1.163Keywords:
artificial intelligence, image processing, effectiveness, artificial visionAbstract
The selection of coffee beans is important for their productivity; however, small or medium-scale producers carry out this process manually, causing limitations that affect their production or marketing. The article aimed to analyze the effectiveness of expert systems in the selection of coffee beans. For this, the study consisted of a systematic review of the literature in the Xplore IEEE, SciencieDirect and SpringerLink databases, of articles published in journals indexed to Scopus, WoS or Scielo; between 2015 and 2021. The result of the review was a matrix of information according to author, article title, techniques or models, and the effectiveness of expert systems. These include the use of RGB image-processing parameters converted to HSV, such as HSL; and the effectiveness of the systems in all cases were greater than 80%. The review concludes that expert systems are effective for the selection of coffee beans, because they optimize time and improve quality in the selection of beans.
Downloads
References
Benet Rodríguez, M., Zafra, S. L., & Quintero Ortega, S. P. (2015). La revisión sistemática de la literatura científica y la necesidad de visualizar los resultados de las investigaciones. Revista Logos, Ciencia & Tecnología, 7(1), 101-103. https://doi.org/10.22335/rlct.v7i1.232
Bonilla Medina, J. (2017). Los beneficios del consumo de café. Revista Facultad Ciencias de la Salud. Universidad del Cauca, 19(2), 47-48. https://revistas.unicauca.edu.co/index.php/rfcs/article/view/177
de Oliveira, E. M., Leme, D. S., Barbosa, B. H. G., Rodarte, M. P., & Pereira, R. G. F. A. (2016). A computer vision system for coffee beans classification based on computational intelligence techniques. Journal of Food Engineering, 171, 22-27. https://doi.org/10.1016/j.jfoodeng.2015.10.009
Flores, W. C., & Pineda, G. M. (2016). A type-2 fuzzy logic system approach to train Honduran coffee cuppers. 2016 IEEE Latin American Conference on Computational Intelligence (LA-CCI), 1-6. https://doi.org/10.1109/LA-CCI.2016.7885710
Gómez-Luna, E., Fernando-Navas, D., Aponte-Mayor, G., & Betancourt-Buitrago, L. A. (2014). Metodología para la revisión bibliográfica y la gestión de información de temas científicos, a través de su estructuración y sistematización. DYNA, 81(184), 1558-163. https://doi.org/10.15446/dyna.v81n184.37066
Hakim, M., Djatna, T., & Yuliasih, I. (2020). Deep Learning for Roasting Coffee Bean Quality Assessment Using Computer Vision in Mobile Environment. 2020 International Conference on Advanced Computer Science and Information Systems (ICACSIS), 363-370. https://doi.org/10.1109/ICACSIS51025.2020.9263224
Herrera, J. C., Medina, S. M., Beleño, K., & Gualdrón, O. E. (2016). Diseño de un sistema automático de selección de frutos de café mediante técnicas de visión artificial. Revista UIS Ingenierías, 15(1), 7-14. https://doi.org/10.18273/revuin.v15n1-2016001
Herrera Pérez, J. C., Medina Ortiz, S. M., Martínez Llano, G. E., Beleño Sáenz, K. de J., & Berrio Pérez, J. S. (2016). Clasificación de los frutos de café según su estado de maduración y detección de la broca mediante técnicas de procesamiento de imágenes. Prospectiva, 14(1), 15. https://doi.org/10.15665/rp.v14i1.640
Huang, N.-F., Chou, D.-L., & Lee, C.-A. (2019). Real-Time Classification of Green Coffee Beans by Using a Convolutional Neural Network. 2019 3rd International Conference on Imaging, Signal Processing and Communication (ICISPC), 107-111. https://doi.org/10.1109/ICISPC.2019.8935644
Janandi, R., & Cenggoro, T. W. (2020). An Implementation of Convolutional Neural Network for Coffee Beans Quality Classification in a Mobile Information System. 2020 International Conference on Information Management and Technology (ICIMTech), 218-222. https://doi.org/10.1109/ICIMTech50083.2020.9211257
Julca-Otiniano, A., Alarcón-Águila, G., Alvarado-Huamán, L., Borjas-Ventura, R., & Castro-Cepero, V. (2018). Comportamiento de tres cultivares de café (catimor, colombia y costa rica 95) en el valle de el Perené, Junín, Perú. Chilean journal of agricultural & animal sciences, 34(ahead), 0-0. https://doi.org/10.4067/S0719-38902018005000504
Junta Nacional del Café. (2021, febrero). Producción y exportaciones de café apuntan a ser mejores durante el 2021. https://juntadelcafe.org.pe/produccion-y-exportaciones-de-cafe-apuntan-a-ser-mejores-durante-el-2021/
Kumar, M., Gupta, P., Madhav, P., & Sachin. (2020). Disease Detection in Coffee Plants Using Convolutional Neural Network. 2020 5th International Conference on Communication and Electronics Systems (ICCES), 755-760. https://doi.org/10.1109/ICCES48766.2020.9138000
Leme, D. S., da Silva, S. A., Barbosa, B. H. G., Borém, F. M., & Pereira, R. G. F. A. (2019). Recognition of coffee roasting degree using a computer vision system. Computers and Electronics in Agriculture, 156, 312-317. https://doi.org/10.1016/j.compag.2018.11.029
Livio, J., Flores, W. C., Hodhod, R., & Umphress, D. (2018). Smart Fuzzy Cupper: Employing approximate reasoning to derive coffee bean quality scoring from individual attributes. 2018 IEEE International Conference on Fuzzy Systems (FUZZ-IEEE), 1-7. https://doi.org/10.1109/FUZZ-IEEE.2018.8491513
Livio, J., & Hodhod, R. (2018). AI Cupper: A Fuzzy Expert System for Sensorial Evaluation of Coffee Bean Attributes to Derive Quality Scoring. IEEE Transactions on Fuzzy Systems, 26(6), 3418-3427. https://doi.org/10.1109/TFUZZ.2018.2832611
Lopes, A. C. A., Andrade, R. P., de Oliveira, L. C. C., Lima, L. M. Z., Santiago, W. D., de Resende, M. L. V., das Graças Cardoso, M., & Duarte, W. F. (2020). Production and characterization of a new distillate obtained from fermentation of wet processing coffee by-products. Journal of Food Science and Technology, 57(12), 4481-4491. https://doi.org/10.1007/s13197-020-04485-4
Monsalve, D., Trujillo, M., & Chaves, D. (2015). Automatic Classification of Nutritional Deficiencies in Coffee Plants. 6th Latin-American Conference on Networked and Electronic Media (LACNEM 2015). https://doi.org/10.1049/ic.2015.0317
Montalbo, F. J. P., & Hernandez, A. A. (2020). An Optimized Classification Model for Coffea Liberica Disease using Deep Convolutional Neural Networks. 2020 16th IEEE International Colloquium on Signal Processing & Its Applications (CSPA), 213-218. https://doi.org/10.1109/CSPA48992.2020.9068683
Ocampo Lopez, O. L., & Alvarez-Herrera, L. M. (2017). Tendencia de la producción y el consumo del café en Colombia. Apuntes CENES, 36(64), 139-165. https://doi.org/10.19053/01203053.v36.n64.2017.5419
Oscco Medina, I., Roldan Ccoycca, E. P., Quispe Murga, E., Camacho Villalobos, A., Marmolejo G., D., & Marmolejo G., K. J. (2020). Selección, identificación y zonificación de café (Coffea arabica L.) por su adaptabilidad, rendimiento, calidad sensorial y resistencia a plagas y enfermedades. Agroindustrial Science, 10(3), 249-257. https://doi.org/10.17268/agroind.sci.2020.03.05
Pham, Y., Reardon-Smith, K., Mushtaq, S., & Cockfield, G. (2019). The impact of climate change and variability on coffee production: a systematic review. Climatic Change, 156(4), 609-630. https://doi.org/10.1007/s10584-019-02538-y
Pinedo-Tuanama, Ll., & Valles-Coral, M. (2021). Importancia de los referenciadores bibliográficos en la gestión de la información científica en tesistas universitarios. Anales de Documentación, 24(2). https://doi.org/10.6018/analesdoc.465091
Portugal-Zambrano, C. E., Gutierrez-Caceres, J. C., Ramirez-Ticona, J., & Beltran-Castanon, C. A. (2016). Computer vision grading system for physical quality evaluation of green coffee beans. 2016 XLII Latin American Computing Conference (CLEI), 1-11. https://doi.org/10.1109/CLEI.2016.7833383
Pradana-López, S., Pérez-Calabuig, A. M., Cancilla, J. C., Lozano, M. Á., Rodrigo, C., Mena, M. L., & Torrecilla, J. S. (2021). Deep transfer learning to verify quality and safety of ground coffee. Food Control, 122, 107801. https://doi.org/10.1016/j.foodcont.2020.107801
Rosas-Echevarría, C., Solís-Bonifacio, H., & Cerna-Cueva, A. (2019). Sistema eficiente y de bajo costo para la selección de granos de café: una aplicación de la visión artificial. Scientia Agropecuaria, 10(3), 347-351. https://doi.org/10.17268/sci.agropecu.2019.03.04
Sanchez-Aguiar, A. F., Mario Ceballos-Arroyo, A., & Espinosa-Bedoya, A. (2019). Toward the recognition of non-defective coffee beans by means of digital image processing. 2019 XXII Symposium on Image, Signal Processing and Artificial Vision (STSIVA), 1-5. https://doi.org/10.1109/STSIVA.2019.8730267
Sandoval Pillajo, A. L., Checa Cabrera, M. A., Díaz Vásquez, R. A., & Acosta Espinoza, J. L. (2021). Sistema experto para el diagnóstico y tratamiento de enfermedades y plagas en plantas ornamentales. Universidad y Sociedad, 13(3), 505-511. https://rus.ucf.edu.cu/index.php/rus/article/view/2127
Serrano Fuentes, M., Lizardo Zelaya, N. A., & Ordoñez Ávila, J. L. (2020). Coffee Fruit Recognition Using Artificial Vision and neural NETWORKS. 2020 5th International Conference on Control and Robotics Engineering (ICCRE), 224-228. https://doi.org/10.1109/ICCRE49379.2020.9096441
Smith Dumont, E., Gassner, A., Agaba, G., Nansamba, R., & Sinclair, F. (2019). The utility of farmer ranking of tree attributes for selecting companion trees in coffee production systems. Agroforestry Systems, 93(4), 1469-1483. https://doi.org/10.1007/s10457-018-0257-z
Sosa, J., Ramirez, J., Vives, L., & Kemper, G. (2019). An Algorithm For Detection of Nutritional Deficiencies from Digital Images of Coffee Leaves Based on Descriptors and Neural Networks. 2019 XXII Symposium on Image, Signal Processing and Artificial Vision (STSIVA), 1-5. https://doi.org/10.1109/STSIVA.2019.8730286
Soto, J., Paiva, E., Ipanaque, W., Reyes, J., Espinoza, D., & Mendoza, D. (2017). Cocoa bean quality assessment by using hyperspectral index for determining the state of fermentation with a non-destructive analysis. 2017 CHILEAN Conference on Electrical, Electronics Engineering, Information and Communication Technologies (CHILECON), 1-5. https://doi.org/10.1109/CHILECON.2017.8229718
Torres Caballero, E. M., & Reyes Duke, A. M. (2020). Implementation of Artificial Neural Networks Using NVIDIA Digits and OpenCV for Coffee Rust Detection. 2020 5th International Conference on Control and Robotics Engineering (ICCRE), 246-251. https://doi.org/10.1109/ICCRE49379.2020.9096435
Vives Garnique, L. A., Mejía Cabrera, H. I., Vilcherrez Chavarry, K. L., & Vassallo Barco, M. J. (2014). Visión artificial: Aplicación de filtros y segmentación en imágenes de hojas de café. Revista INGENIERÍA: Ciencia Tecnología e Innovación, 1(2), 71-81. http://revistas.uss.edu.pe/index.php/ING/article/view/198
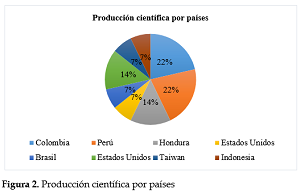
Published
How to Cite
Issue
Section
License
Copyright (c) 2022 Merly Monsalve-Vásquez

This work is licensed under a Creative Commons Attribution 4.0 International License.