Prediction of student academic performance using supervised learning algorithms in a university from the Peruvian rainforest
DOI:
https://doi.org/10.55873/rad.v3i1.292Keywords:
data analysis, educational prediction, machine learning, data miningAbstract
Academic performance is crucial for educational management and predicting it can optimize decision-making processes. This study aimed to predict the academic performance of university students using three supervised learning algorithms: K-Nearest Neighbors (KNN), Naive Bayes (NB), and Decision Tree (AD). Data from 813 students, including socioeconomic and academic variables, were processed and evaluated using metrics such as precision, recall, accuracy, and AUC-ROC. The KNN model was the most effective, achieving an accuracy of 81.97%, making it the best choice for predicting academic performance. Although it showed moderate recall, its precision was the highest, demonstrating a good balance in student classification. In conclusion, KNN is a promising tool for educational institutions to identify at-risk students and improve intervention strategies, helping to boost academic performance and reduce dropout rates.
Downloads
References
Abelha, M., Fernandes, S., Mesquita, D., Seabra, F., & Ferreira-Oliveira, A. T. (2020). Graduate Employability and Competence Development in Higher Education—A Systematic Literature Review Using PRISMA. Sustainability, 12(15), 5900. https://doi.org/10.3390/su12155900
Bañeres, D., Rodríguez-González, M. E., Guerrero-Roldán, A.-E., & Cortadas, P. (2023). An early warning system to identify and intervene online dropout learners. International Journal of Educational Technology in Higher Education, 20(1), 3. https://doi.org/10.1186/s41239-022-00371-5
Centoni, M., & Maruotti, A. (2021). Students’ evaluation of academic courses: An exploratory analysis to an Italian case study. Studies in Educational Evaluation, 70, 101054. https://doi.org/10.1016/j.stueduc.2021.101054
Goss, H. (2022). Student Learning Outcomes Assessment in Higher Education and in Academic Libraries: A Review of the Literature. The Journal of Academic Librarianship, 48(2), 102485. https://doi.org/10.1016/j.acalib.2021.102485
Haleem, A., Javaid, M., Qadri, M. A., & Suman, R. (2022). Understanding the role of digital technologies in education: A review. Sustainable Operations and Computers, 3, 275–285. https://doi.org/10.1016/j.susoc.2022.05.004
Issah, I., Appiah, O., Appiahene, P., & Inusah, F. (2023). A systematic review of the literature on machine learning application of determining the attributes influencing academic performance. Decision Analytics Journal, 7, 100204. https://doi.org/10.1016/j.dajour.2023.100204
Johar, N. A., Kew, S. N., Tasir, Z., & Koh, E. (2023). Learning Analytics on Student Engagement to Enhance Students’ Learning Performance: A Systematic Review. Sustainability, 15(10), 7849. https://doi.org/10.3390/su15107849
Kamalov, F., Santandreu Calonge, D., & Gurrib, I. (2023). New Era of Artificial Intelligence in Education: Towards a Sustainable Multifaceted Revolution. Sustainability, 15(16), 12451. https://doi.org/10.3390/su151612451
Matz, S. C., Bukow, C. S., Peters, H., Deacons, C., Dinu, A., & Stachl, C. (2023). Using machine learning to predict student retention from socio-demographic characteristics and app-based engagement metrics. Scientific Reports, 13(1), 5705. https://doi.org/10.1038/s41598-023-32484-w
Matzavela, V., & Alepis, E. (2021). Decision tree learning through a Predictive Model for Student Academic Performance in Intelligent M-Learning environments. Computers and Education: Artificial Intelligence, 2, 100035. https://doi.org/10.1016/j.caeai.2021.100035
Nsanzumuhire, S. U., & Groot, W. (2020). Context perspective on University-Industry Collaboration processes: A systematic review of literature. Journal of Cleaner Production, 258, 120861. https://doi.org/10.1016/j.jclepro.2020.120861
Núñez-Canal, M., de Obesso, M. de las M., & Pérez-Rivero, C. A. (2022). New challenges in higher education: A study of the digital competence of educators in Covid times. Technological Forecasting and Social Change, 174, 121270. https://doi.org/10.1016/j.techfore.2021.121270
Rahman, S., Munam, A. M., Hossain, A., Hossain, A. S. M. D., & Bhuiya, R. A. (2023). Socio-economic factors affecting the academic performance of private university students in Bangladesh: a cross-sectional bivariate and multivariate analysis. SN Social Sciences, 3(2), 26. https://doi.org/10.1007/s43545-023-00614-w
Sghir, N., Adadi, A., & Lahmer, M. (2023). Recent advances in Predictive Learning Analytics: A decade systematic review (2012–2022). Education and Information Technologies, 28(7), 8299–8333. https://doi.org/10.1007/s10639-022-11536-0
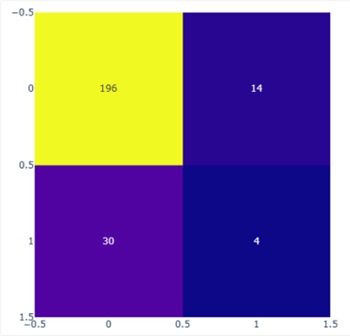
Downloads
Published
How to Cite
Issue
Section
License
Copyright (c) 2024 Alex Ali Vargas-Quispe, Jaime Cesar Prieto-Luna

This work is licensed under a Creative Commons Attribution 4.0 International License.