Predicción del rendimiento académico estudiantil usando algoritmos de aprendizaje supervisado en una universidad de la selva peruana
DOI:
https://doi.org/10.55873/rad.v3i1.292Palabras clave:
análisis de datos, aprendizaje automático, minería de datos, predicción educativaResumen
El rendimiento académico es crucial para la gestión educativa, y la predicción de este puede optimizar la toma de decisiones. Este estudio tuvo como objetivo predecir el rendimiento académico de estudiantes universitarios mediante tres algoritmos de aprendizaje supervisado: K-Vecinos más cercanos (KNN), Naive Bayes (NB) y Árbol de Decisión (AD). Se utilizaron datos de 813 estudiantes, incluyendo variables socioeconómicas y académicas. Los datos fueron preprocesados y evaluados a través de métricas como precisión, recuperación, exactitud y AUC-ROC. El modelo KNN fue el más efectivo, alcanzando una exactitud del 81.97%, lo que lo convierte en la mejor opción para predecir el rendimiento académico. Aunque mostró una recuperación moderada, su precisión fue la más alta, demostrando un buen equilibrio en la clasificación de los estudiantes. En conclusión, KNN es una herramienta prometedora para que las instituciones educativas identifiquen estudiantes en riesgo y mejoren sus estrategias de intervención, ayudando a elevar el rendimiento académico y reducir la deserción.
Descargas
Citas
Abelha, M., Fernandes, S., Mesquita, D., Seabra, F., & Ferreira-Oliveira, A. T. (2020). Graduate Employability and Competence Development in Higher Education—A Systematic Literature Review Using PRISMA. Sustainability, 12(15), 5900. https://doi.org/10.3390/su12155900
Bañeres, D., Rodríguez-González, M. E., Guerrero-Roldán, A.-E., & Cortadas, P. (2023). An early warning system to identify and intervene online dropout learners. International Journal of Educational Technology in Higher Education, 20(1), 3. https://doi.org/10.1186/s41239-022-00371-5
Centoni, M., & Maruotti, A. (2021). Students’ evaluation of academic courses: An exploratory analysis to an Italian case study. Studies in Educational Evaluation, 70, 101054. https://doi.org/10.1016/j.stueduc.2021.101054
Goss, H. (2022). Student Learning Outcomes Assessment in Higher Education and in Academic Libraries: A Review of the Literature. The Journal of Academic Librarianship, 48(2), 102485. https://doi.org/10.1016/j.acalib.2021.102485
Haleem, A., Javaid, M., Qadri, M. A., & Suman, R. (2022). Understanding the role of digital technologies in education: A review. Sustainable Operations and Computers, 3, 275–285. https://doi.org/10.1016/j.susoc.2022.05.004
Issah, I., Appiah, O., Appiahene, P., & Inusah, F. (2023). A systematic review of the literature on machine learning application of determining the attributes influencing academic performance. Decision Analytics Journal, 7, 100204. https://doi.org/10.1016/j.dajour.2023.100204
Johar, N. A., Kew, S. N., Tasir, Z., & Koh, E. (2023). Learning Analytics on Student Engagement to Enhance Students’ Learning Performance: A Systematic Review. Sustainability, 15(10), 7849. https://doi.org/10.3390/su15107849
Kamalov, F., Santandreu Calonge, D., & Gurrib, I. (2023). New Era of Artificial Intelligence in Education: Towards a Sustainable Multifaceted Revolution. Sustainability, 15(16), 12451. https://doi.org/10.3390/su151612451
Matz, S. C., Bukow, C. S., Peters, H., Deacons, C., Dinu, A., & Stachl, C. (2023). Using machine learning to predict student retention from socio-demographic characteristics and app-based engagement metrics. Scientific Reports, 13(1), 5705. https://doi.org/10.1038/s41598-023-32484-w
Matzavela, V., & Alepis, E. (2021). Decision tree learning through a Predictive Model for Student Academic Performance in Intelligent M-Learning environments. Computers and Education: Artificial Intelligence, 2, 100035. https://doi.org/10.1016/j.caeai.2021.100035
Nsanzumuhire, S. U., & Groot, W. (2020). Context perspective on University-Industry Collaboration processes: A systematic review of literature. Journal of Cleaner Production, 258, 120861. https://doi.org/10.1016/j.jclepro.2020.120861
Núñez-Canal, M., de Obesso, M. de las M., & Pérez-Rivero, C. A. (2022). New challenges in higher education: A study of the digital competence of educators in Covid times. Technological Forecasting and Social Change, 174, 121270. https://doi.org/10.1016/j.techfore.2021.121270
Rahman, S., Munam, A. M., Hossain, A., Hossain, A. S. M. D., & Bhuiya, R. A. (2023). Socio-economic factors affecting the academic performance of private university students in Bangladesh: a cross-sectional bivariate and multivariate analysis. SN Social Sciences, 3(2), 26. https://doi.org/10.1007/s43545-023-00614-w
Sghir, N., Adadi, A., & Lahmer, M. (2023). Recent advances in Predictive Learning Analytics: A decade systematic review (2012–2022). Education and Information Technologies, 28(7), 8299–8333. https://doi.org/10.1007/s10639-022-11536-0
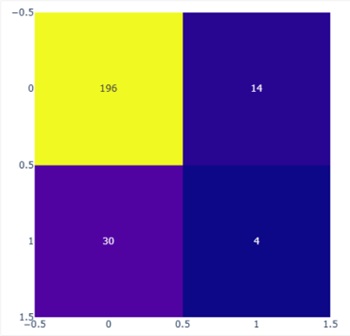
Descargas
Publicado
Cómo citar
Número
Sección
Licencia
Derechos de autor 2024 Alex Ali Vargas-Quispe, Jaime Cesar Prieto-Luna

Esta obra está bajo una licencia internacional Creative Commons Atribución 4.0.