Análisis de datos y pronóstico de casos de la Covid-19 en el departamento de Madre de Dios de Perú utilizando técnicas LSTM
DOI:
https://doi.org/10.55873/rad.v1i2.195Palabras clave:
Covid-19, LSTM, infectados, pronóstico, pandemiaResumen
Actualmente la Covid-19 está causando grandes pérdidas a nivel mundial, es por ello que diferentes trabajos permiten predecir o pronosticar el comportamiento de cantidad de infectados utilizando técnicas de forecasting, dentro del campo de Inteligencia Artificial se están permitiendo tomar medidas de control en los diferentes países. En este trabajo se propuso un modelo de aprendizaje profundo para pronosticar los casos diarios en las regiones de Madre de Dios. Los datos utilizados pertenecen al conjunto de datos abiertos Covid-19 del Ministerio de Salud de Perú (MINSA). El conjunto de datos incluye los períodos de inicio de marzo de 2020 a fines de diciembre de 2021. Se utilizó un LSTM utilizando variables de Fecha, Departamento, Provincia, Distrito, Casos, IP. ID y con tamaño de ventana de 5 días, se obtuvo una precisión de 94,67% con los datos de entrenamiento y un 92,31%.
Descargas
Citas
Aguilar I., L., Ibáñez-Reluz, M., Z. Aguilar, J. C., Zavaleta-Aguilar, E. W., & Aguilar, L. A. (2021). Forecasting SARS-CoV-2 in the peruvian regions: a deep learning approach using temporal convolutional neural networks. Selecciones Matemáticas, 8(1), 12–26. https://doi.org/10.17268/sel.mat.2021.01.02
Arora, P., Kumar, H., & Panigrahi, B. K. (2020). Prediction and analysis of COVID-19 positive cases using deep learning models: A descriptive case study of India. Chaos, Solitons and Fractals Fractals, 139, 110017. https://doi.org/10.1016/j.chaos.2020.110017
Ayyoubzadeh, S. M., Ayyoubzadeh, S. M., Zahedi, H., Ahmadi, M., & R Niakan Kalhori, S. (2020). Predicting COVID-19 Incidence Through Analysis of Google Trends Data in Iran: Data Mining and Deep Learning Pilot Study. JMIR Public Health and Surveillance, 6(2), e18828. https://doi.org/10.2196/18828
Cocconi, M., & Roark, G. (2020). Predicción de contagios, recuperaciones y casos fatales de COVID-19 en Argentina a través del uso de modelos de regresión no lineal como base para la planificación de recursos hospitalarios. XIII COINI 2020 UTN FRBA - Congreso Argentino Internacional de Ingeniería Industrial.
Cruz-Mendoza, I., Quevedo-Pulido, J., & Adanaque-Infante, L. (2020). LSTM perfomance analysis for predictive models based on Covid-19 dataset. 2020 IEEE XXVII International Conference on Electronics, Electrical Engineering and Computing (INTERCON), 1–4. https://doi.org/10.1109/INTERCON50315.2020.9220248
Fanelli, D., & Piazza, F. (2020). Analysis and forecast of COVID-19 spreading in China, Italy and France. Chaos, Solitons and Fractals, 134, 109761. https://doi.org/10.1016/j.chaos.2020.109761
Franco, E. F., Calderón, V. V., & Ramos, R. T. (2020). Modelos de predicción del impacto y evolución del COVID-19 en República Dominicana. Ciencia, Ambiente y Clima, 3(1), 5–21. https://doi.org/10.22206/cac.2020.v3i1.pp5-21
Garrido, J. M., Martínez-Rodríguez, D., Rodríguez-Serrano, F., Pérez-Villares, J. M., Ferreiro-Marzal, A., Jiménez-Quintana, M. M., & Villanueva, R. J. (2022). Mathematical model optimized for prediction and health care planning for COVID-19. Medicina Intensiva (English Edition), 46(5), 248–258. https://doi.org/10.1016/j.medine.2022.02.020
Kafieh, R., Arian, R., Saeedizadeh, N., Amini, Z., Serej, N. D., Minaee, S., Yadav, S. K., Vaezi, A., Rezaei, N., & Haghjooy Javanmard, S. (2021). COVID-19 in Iran: Forecasting Pandemic Using Deep Learning. Computational and Mathematical Methods in Medicine, 2021, 1–16. https://doi.org/10.1155/2021/6927985
Khakharia, A., Shah, V., Jain, S., Shah, J., Tiwari, A., Daphal, P., Warang, M., & Mehendale, N. (2021). Outbreak Prediction of COVID-19 for Dense and Populated Countries Using Machine Learning. Annals of Data Science, 8(1), 1–19. https://doi.org/10.1007/s40745-020-00314-9
Lalmuanawma, S., Hussain, J., & Chhakchhuak, L. (2020). Applications of machine learning and artificial intelligence for Covid-19 (SARS-CoV-2) pandemic: A review. Chaos, Solitons & Fractals, 139, 110059. https://doi.org/10.1016/j.chaos.2020.110059
Mendieta, J. F. M., Cortes Cortes, M. E., Cortes Iglesias, M., Perez Fernandez, A. del C., & Manzano Cabrera, M. (2020). Study on predictive models for COVID-19 in Cuba. Medisur-Revista De Ciencias Medicas De Cienfuegos, 18(3), 431–442. https://pesquisa.bvsalud.org/global-literature-on-novel-coronavirus-2019-ncov/resource/pt/grc-741565
Shinde, G. R., Kalamkar, A. B., Mahalle, P. N., Dey, N., Chaki, J., & Hassanien, A. E. (2020). Forecasting Models for Coronavirus Disease (COVID-19): A Survey of the State-of-the-Art. SN Computer Science, 1(4), 197. https://doi.org/10.1007/s42979-020-00209-9
Sujath, R., Chatterjee, J. M., & Hassanien, A. E. (2020). A machine learning forecasting model for COVID-19 pandemic in India. Stochastic Environmental Research and Risk Assessment, 34(7), 959–972. https://doi.org/10.1007/s00477-020-01827-8
Wang, P., Zheng, X., Ai, G., Liu, D., & Zhu, B. (2020). Time series prediction for the epidemic trends of COVID-19 using the improved LSTM deep learning method: Case studies in Russia, Peru and Iran. Chaos, Solitons and Fractals, 140, 110214. https://doi.org/10.1016/j.chaos.2020.110214
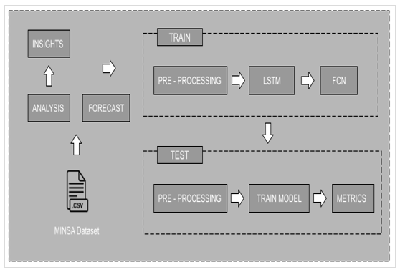
Publicado
Cómo citar
Número
Sección
Licencia
Derechos de autor 2022 Jose Carlos Navarro-Vega, Nelly Jacqueline Ulloa-Gallardo, Diego Raphael Paz-Bustamante, Diego Gustavo Zegarra-Conde, Wilder Nina-Choquehuayta

Esta obra está bajo una licencia internacional Creative Commons Atribución 4.0.